Although computed tomography (CT) screening has emerged as an essential tool for the early detection of lung diseases, there is still a broad range of potential improvements to be made. According to recent research, understanding the patterns of onset for earlier prediction of disease may be achieved by leveraging large databases and more advanced technologic solutions such as artificial intelligence (AI).
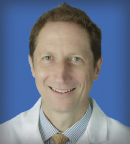
R. Graham Barr, MD, DrPH
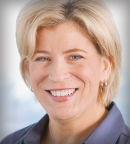
Elizabeth Estes
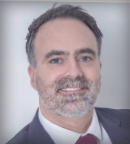
Simon Walsh, MD, PhD
In a panel discussion presented during Prevent Cancer Foundation’s Quantitative Imaging Workshop XX, R. Graham Barr, MD, DrPH; Elizabeth Estes; and Simon Walsh, MD, PhD, shared their thoughts on the role of machine learning and AI to identify and treat lung diseases while cautioning the need for careful handling of data acquired from lung cancer screening.1
Non–Lung Cancer Findings
The moderator of the session, Albert Rizzo, MD, FCCP, FACP, FAASM, of the American Lung Association, emphasized that although CT scans have revolutionized the detection of lung cancer and significantly reduced lung cancer mortality figures, the impact of screening is no longer limited to lung cancer alone.
“Non–lung cancer findings derived from low-dose CT scans have become indispensable in helping clinicians make early interventions aimed at preserving the health of tobacco-exposed patients,” said Dr. Rizzo, who noted the American College of Radiology has a database of more than 4.5 million scans with noncancerous findings related to tobacco exposure. “Routine screening of thoracic images now reveals the presence of early emphysema, interstitial lung changes, and coronary calcifications.”
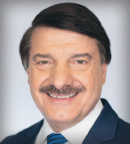
Albert Rizzo, MD, FCCP, FACP, FAASM
According to Dr. Rizzo, findings about emphysema may be acting as a radiographic biomarker of an individual who is at risk for the development of symptomatic, clinically relevant obstructive airways disease, and findings of interstitial changes may be similar markers. Although there are currently no specific interventions or modifying agents available for emphysema, said Dr. Rizzo, health-care providers may still be able to act on this information.
Predicting Disease Trajectories
Dr. Barr, Chief of General Medicine at Columbia University, New York, focuses his research on the epidemiology and causes of chronic lower respiratory diseases. He noted that studies have shown an increased percentage of emphysematous voxels (small units of a three-dimensional image on a CT scan related to areas of lung damage) is associated with a 2.5-fold increased hazard ratio of death. According to Dr. Barr, this factor is even greater in people with a history of smoking.
The presence of emphysema also indicated an increased rate of death from lung disease, lung cancer, and cardiovascular disease, said Dr. Barr, who recommended checking for spirometer-defined chronic obstructive pulmonary disease (COPD) in patients with emphysema, in addition to smoking cessation. Dysanapsis (disproportionate scaling of airway dimensions to lung volume) is another consideration that may be derived from lung cancer screening CT scans and is a significant risk factor for COPD.
Dr. Barr and colleagues are currently using deep learning–based approaches to analyze images of more than 20,000 participants in cohorts of varying phenotypes. However, Dr. Barr also stressed the need for vigilance against “artificial stupidity.”
“Things can go wrong with AI, and they go wrong very badly and very quickly,” Dr. Barr observed. “Minor adjustments in scanner or algorithm may have huge consequences in terms of results. We need to be mindful and do rigorous science to make sure things are really working.”
Open-Source Imaging Consortium
The Open-Source Imaging Consortium (OSIC) is also making significant strides in analyzing parenchymal lung diseases using machine learning and deep learning algorithms. With Ms. Estes from OSIC, Dr. Walsh, of the National Lung and Heart Institute, Imperial College London, United Kingdom, highlighted the efforts taken to standardize automatic image analysis for patients with interstitial lung disease. As part of their initiative, they have curated and integrated the largest and most diverse interstitial lung disease imaging data set, with more than 5,000 scans.
In their discussion, Dr. Walsh and Ms. Estes noted the limitations of standard tests such as forced vital capacity, which is characterized by measurement variability and is prone to missing data, in measuring disease progression in fibrotic lung disease. However, CT scans open a world of opportunities for more sensitive and reliable measurements. As Dr. Walsh explained, CT is central to the diagnosis of fibrotic lung disease; can be used to predict outcomes and monitor disease progression response to treatment; and is central to eligibility assessments for drug trial enrollment. Although CT scans are associated with high levels of intraobserver variability, the large data sets being collected and harmonized by OSIC will lead to deeper clinical understanding of progressive lung complications with the help of deep learning algorithms.
“Our goal is to drive more longitudinal scans, because we’ve realized their importance for these algorithms, and we’re starting to add more forms of data,” said Dr. Walsh, who noted lung diseases “sound differently.”
Finally, apart from data collection and analytics, Dr. Walsh underscored the need for adopting a different diagnostic paradigm for fibrotic lung diseases. The current standard practice involves waiting for symptoms to appear, performing a CT scan, and identifying fibrosis, explained Dr. Simon. However, by the time disease has been detected, lung function has already declined significantly.
“With the help of AI, we can shift the focus toward risk stratification to figure out which patients might need more frequent screening and which populations are at very low risk,” Dr. Walsh explained. “This will hopefully lead to earlier diagnoses and treatment interventions.”
Dr. Walsh and colleagues will have 10,000 lung cancer screens added to their database by the end of this year, with a goal of 100,000 in 2024.
DISCLOSURE: All speakers reported no conflicts of interest.
REFERENCE