Artificial intelligence (AI) has captured society’s imagination and generated enthusiasm for its potential to improve our quality of life, especially in the health-care arena. The availability of high-dimensionality data sets along with innovations in high-performance computing and deep-learning architectures has created an explosion of AI use in various aspects of oncology; these uses range from detection and classification of cancer to molecular characterization of tumors and their microenvironment, leading to drug discovery and predicting treatment outcomes for patients. Yet, although AI is rapidly being incorporated into oncologic research, work remains to be done to translate these studies into real-world, clinically meaningful applications.
Artificial Intelligence: A Tool, Not a Threat
According to the genitourinary oncologist Pedro Barata, MD, MSc, Assistant Professor at Tulane University Medical School, New Orleans, “AI technologies must pass through several hurdles before finding their way into the clinical oncology setting. Beyond their technical development, they must be trained on high-quality, annotated data sets that capture the rich diversity of the target patient population. AI products are then subject to rigorous premarket review by the FDA through its medical device regulatory framework. Once cleared or ‘approved’ by the agency, they must be integrated within departmental workflow patterns. In other words, how AI tools are utilized in clinical practice largely depends on internal protocols and the way physicians are trained in their use. Lastly, reimbursement of AI products remains an emerging issue; the Centers for Medicare & Medicaid Services has moved to create AI-specific CPT codes only within the past year.”
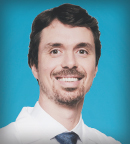
Pedro Barata, MD, MSc
Although AI faces adoption challenges, Dr. Barata noted that it has demonstrated accurate technical performance in image analysis, predictive analytics, and several emerging aspects in the delivery of precision oncology. However, he also offered a caveat: the performance of AI in clinical care relies on data.
“Put simply, AI algorithms are only as good as the data they train on. The quality of their output should scale with the increasing availability and robustness of clinical data,” explained Dr. Barata.
Liability Issues
As we enter new uncharted terrain with AI, there are some potential liability issues to be identified. Vrushab Gowda, JD, a fellow at Harvard’s Petrie-Flom Center for Health Law Policy, who has published on AI in oncology with Dr. Barata, commented: “There are a number of liability issues on the horizon. For one, reliance upon erroneous AI output (ie, missed findings or false-positive/negative diagnoses) straightforwardly implicates liability. The flip side invites its own challenges; if physicians substitute a correct AI readout and with their own false clinical judgment, they may find themselves exposed to malpractice risk. The quanta of risk may grow over time as AI integrates into evidence-based guidelines or becomes standard clinical practice. Additionally, a host of informed consent issues, for both patients and those whose health information are used in training data sets, lurk in the background.”
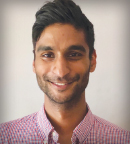
Vrushab Gowda, JD
As with any transformative technology, studies indicate an undercurrent of unease about the heretofore unknown role AI will play in the future of oncology, especially in the unique doctor-patient relationship. However, Mr. Gowda stressed that AI technologies such as machine learning can profoundly optimize the existing mode of anticancer drug research and treatment, but AI also has its relative limitation and should be viewed as a tool, not a threat.
Mr. Gowda continued: “Physicians should not feel alarmed by the emergence of AI, but rather treat it as one among many novel clinical tools at their disposal. Machines will not supplant human physicians any time soon or threaten their job security; technical, regulatory, legal, and practical constraints impose sharp limitations on their autonomous clinical decision-making capabilities.”
Artificial Intelligence and Precision Medicine
Studies have shown that the intersection of genomics, precision medicine, and AI may help us develop better predictive markers for drug development. Olivier Elemento, PhD, Director of the Weill Cornell Englander Institute for Precision Medicine, shared these comments with The ASCO Post: “We’re entering an era in which the response to specific treatments is not based on a single marker but
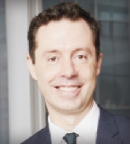
Olivier Elemento, PhD
rather on multiple features; for instance, tumor mutation burden, which predicts response to checkpoint inhibitors, is defined as the number of somatic mutations per megabase of interrogated genomic sequence, and it varies across malignancies. But even that knowledge is not always enough to predict response, as we need additional biomarkers from patients, such as presence or absence of certain driver mutations and the activity, abundance, and localization of certain types of immune cells. Technologies such as whole-genome sequencing and imaging mass cytometry can be used simultaneously to quantify thousands of such biomarkers in tumor samples. Increasingly, AI will be used to combine all this pertinent information into algorithms that accurately predict therapy -responses.”
Epigenetics
In today’s complex mix of therapies that are offered to a varied demographic, oncologists and pathologists must work in concert to target the right patient for the right therapy at the right time. According to Dr. Elemento, given our ability to sequence a patient’s entire cancer genome in just a few days, molecular predictive testing is undergoing a rapid evolution and increasingly embracing AI.
“We have a tremendous amount of information to be matched with specific treatments in specific patient populations. To that end, AI can be harnessed to support the challenges in this complex process. And, although AI is a valuable tool in decision support, it will not replace the clinical interplay between the pathologist and the cancer management team. It will simply make the process more streamlined and more effective,” said Dr. Elemento.
One area where AI may have the highest impact is epigenetics. Epigenetic changes are present in all human cancers and are now known to cooperate with genetic alterations to drive the cancer phenotype. Dr. Elemento noted that the application of AI to epigenomic studies could significantly enhance diagnostic accuracy and boost patient stratification.
“We are seeing a very interesting trend,” Dr. Elemento explained. “For example, epigenetic profiling in brain tumors is becoming a key component in the diagnosis process. If we just examine tissue morphology, we are not getting the whole clinical picture. However, when you look at the DNA methylation that keys into specific markers in the epigenetic genome, it allows for a much more precise diagnosis. In fact, up to 20% of brain tumors cannot be accurately classified by morphology alone, and we need DNA methylation to make these clinical classifications. This process can reclassify low-grade tumors to high-grade tumors, and vice versa, which naturally alters the treatment decision. Because DNA methylation profiling involves analyzing millions of data points, AI plays a critical role in classifying patients in the general landscape of the disease and places them in the correct group with the right treatment.”
Bringing AI Into the Clinic
Although AI-driven machine-learning algorithms are being used by researchers and clinicians in academic oncology, most of clinical care takes place in the community setting, where AI tools have not gained traction in the care model.
“We still have a long way to go to bridge the gap between the amazing AI tools we have in the academic research lab and the community practices,” commented Dr. Elemento. “We need a concerted effort to address this shortcoming, as AI would really help super-busy clinicians streamline their management models. One of the major hurdles is the technical challenge of integration into the EMR data systems in the community practices. It’s a complex process that needs all stakeholders on board, but once we fully integrate AI tools into the cancer delivery system, it will be transformative.”
DISCLOSURE: Dr. Barata has served as a consultant to Astellas, Eisai, EMD Serono, Dendreon, Pfizer, and Seattle Genetics; has contracted research with AstraZeneca, Merck, Natera, and Caris; has received a research grant from BlueEarth Diagnostics; and has served on a speakers bureau with Bayer and Caris. Mr. Gowda is employed by Give Legacy, Inc. Dr. Elemento has served as a scientific advisor and equity holder in Freenome, Owkin, Volastra Therapeutics, and OneThree Biotech.